Navigating AI Ethics: Tackling Bias, Privacy, and Transparency Issues
Explore the critical issues in AI ethics, including bias, privacy, and transparency. Learn how to address these challenges to ensure ethical and responsible AI development.
Navigating AI Ethics: Tackling Bias, Privacy, and Transparency Issues
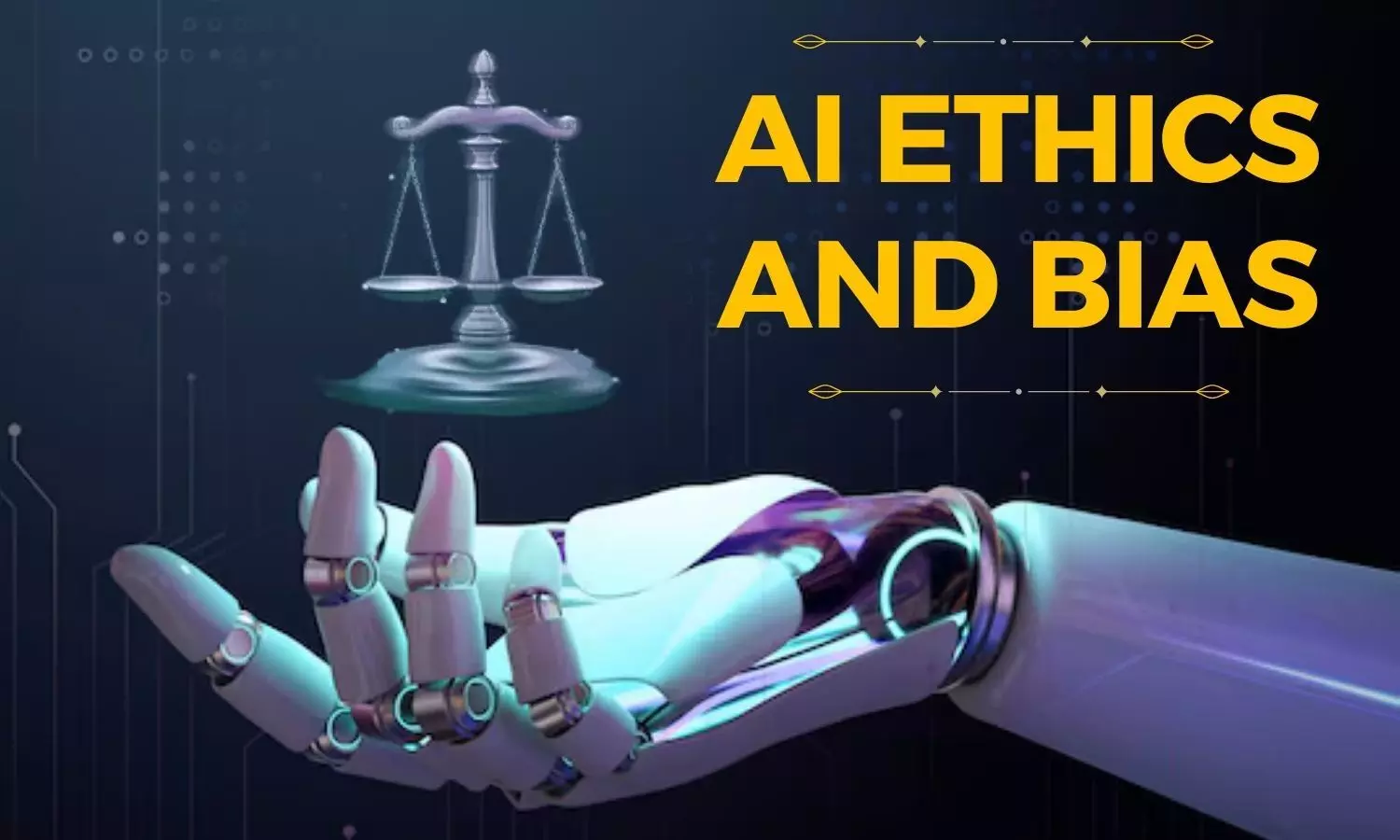
Introduction
Despite the impressive capabilities of artificial intelligence (AI), it's important to remember that these systems are fundamentally just tools. They lack the type of intelligence we humans possess, and specifically, with Large Language Models (LLMs)—the focus of this article—they essentially generate variations of the data they’ve been trained on.
A significant aspect missing from these AI tools is a sense of ethics. While they are trained on a vast array of content from the internet, they do not possess the human ability to discern between well-written content and material that may be ethically questionable.
In 2024, the focus on AI ethics has intensified as we work to tackle bias in AI systems and ensure fairness in AI applications. Developing comprehensive ethical AI guidelines is vital for advancing AI accountability and transparency. Key priorities include mitigating bias in machine learning and establishing robust frameworks for ethical AI practices. By emphasising these areas, we can build ethical AI frameworks that uphold fairness and integrity in technological progress.
Understanding AI Ethics
The importance of ethics in artificial intelligence (AI) has become increasingly prominent in our rapidly evolving technological landscape. Integrating ethical principles into AI is essential to ensure that the content generated reflects both organisational values and broader societal standards.
AI ethics encompasses a wide range of issues, including data responsibility, privacy, fairness, transparency, environmental sustainability, inclusion, accountability, trust, and the potential misuse of technology.
Often, ethical breaches occur unintentionally. When a tool's primary objective is to enhance commercial outcomes, oversight and unintended consequences may arise, particularly due to inadequate initial research and biased datasets. As with any emerging technology, unforeseen risks can emerge. With regulatory frameworks lagging, the responsibility for addressing ethical considerations falls to the creators of new AI systems.
The Need for Transparency
There is growing concern that creators of leading AI technologies must increase transparency about their tools. For example, there is a push for them to disclose the data on which large language models (LLMs) are trained.
Transparency should be maintained throughout all stages of a project’s development, extending beyond just the developers who build the code. It’s crucial to clarify the functions and decision-making processes of AI systems so we can better understand inherent biases and find ways to mitigate them.
Bias and Fairness in AI
Algorithmic bias in AI systems arises when these systems produce results that are systematically prejudiced due to flawed assumptions or limitations in the machine learning process. As AI tools become more prevalent in generating content, it is crucial to understand and apply AI ethics to ensure trust and accountability.
Addressing these biases is essential to foster confidence in AI technologies, particularly in areas where AI decisions have significant consequences, such as job recruitment, credit scoring, healthcare, finance, and law enforcement. Without this understanding, there is a risk of unfair outcomes and erosion of trust.
Some AI systems have already demonstrated problematic results, particularly when their outputs are accepted without critical human review. For instance, research has shown that autonomous driving systems are 20% less effective at recognising children compared to adults and 7.5% less accurate with darker-skinned pedestrians than lighter-skinned ones. This discrepancy is attributed to the biases present in the image data used for training these models.
Another example of inherent biases is seen in image generation tools, which often reflect the unconscious biases present in their training data. For instance, Stable Diffusion's text-to-image model has been found to favour white-skinned men over people of colour and women.
Mitigating and Preventing Bias in AI
To address and prevent algorithmic bias, it is crucial to utilise diverse and representative datasets during the AI training process and to conduct regular audits to identify and correct biases. Achieving this requires the transparency previously discussed, as the consequences of bias are significant.
In 2021, UNESCO introduced the first global standard on AI ethics, emphasising the need for human oversight in AI systems. This framework prioritises the protection of human rights and dignity, focusing specifically on preventing the perpetuation of existing biases.
Even if transparency within some larger organisations progresses slowly, it is essential that AI development involves diverse and multidisciplinary teams to ensure that a wide range of perspectives is considered.
Privacy and Data Protection
AI technologies often depend on vast amounts of data, making it essential to handle personal data responsibly and securely. Mishandling or misuse of this data can lead to privacy breaches and erode trust. When developing systems that utilise customer data, it is crucial to implement stringent data protection measures such as encryption and anonymisation. Without these safeguards, there is an increased risk of personal data being exposed through the AI system’s outputs.
Ideally, AI systems should be designed with privacy as a core principle, collecting and using only the minimum necessary data. This approach not only meets legal requirements in many regions but also plays a vital role in maintaining public trust in your organisation.
AI Guidelines and Regulations
Current regulations and guidelines, while addressing key issues such as privacy, transparency, accountability, and fairness, often lack comprehensiveness specifically for AI use cases. For example, the European Union's General Data Protection Regulation (GDPR) imposes strict rules for data protection and privacy, which extend to AI systems, even though it does not have specific provisions for AI.
Additionally, guidelines from organisations like the OECD and IEEE highlight principles such as transparency, fairness, and human oversight in AI systems. However, due to the rapid advancement of AI technologies, these guidelines and regulations frequently lag and require continuous updates and practical enforcement mechanisms to remain effective.
As we await comprehensive legislation and global protections to address AI's potential harms, organisations should avoid cutting corners. Adopting a humanistic approach to technology benefits businesses and society as a whole, fostering the trust necessary for widespread acceptance and adoption.